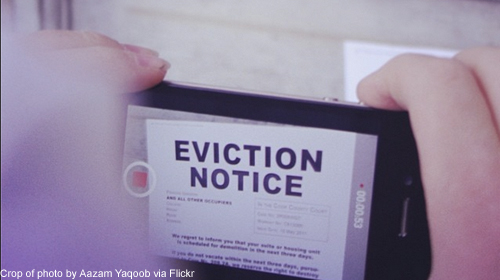
A data analytics company a variety of information about individuals in New York City, including demographic data, court records, employment status, education level, age, and any history of interaction with the foster care system or use of homeless shelters (acquired from New York’s Department of Homeless Services). Using a process its CEO compares to a “highly targeted marketing campaign trying to sell something,” the company then analyzes that data to come up with a list of the 30 to 50 people to be targeted for special attention.
In this case, the population that is examined are the roughly 5,000 people each month who receive eviction notices in New York City. The company, a nonprofit called SumAll, uses the analytics to try to predict which of those people are most likely to become homeless as a result of their evictions, and those who are identified receive personalized letters, hotline numbers and other outreach, and are given priority access to eviction counseling by social services workers.
It may be big, bad “big data,” but that doesn’t sound so terrible. What are we to make of this kind of use of analytics?
This system, which is just a pilot project for now, is detailed in reporting yesterday from . In some ways it raises the same issues as the Chicago Police Department’s use of analytics to create a “heat list” of the “most dangerous people in Chicago.” The people so identified received visits from police officers. As I said about that program, there can be a fine line between laudable efforts to identify and help “at-risk youth,” and efforts to tag some people with labels used to discriminate and stigmatize. A key question about the Chicago program is whether being flagged leads to benefits for a person, like support, opportunities, and increased chances to escape crime, or—as there are all too many reasons to believe—sanctions, such as surveillance and prejudicial encounters with the police.
Here we have an example where the consequences of being flagged by these analytics appear to be pretty clearly positive for individuals, so it provides a cleaner example by which to figure out what we think about such uses. I have several initial thoughts about this.
First, the consequences of data mining matter. Big data is a concern because we worry that it will lead to bad things for many people, such as injustice, and that on a broader social level it will further tilt power from the weak to the strong and increase divisions between the haves and have-nots. But where big data analytics is used for purposes that improve people’s lives, that is important.
Of course, even if the immediate consequences of data mining are helpful for its subjects, it is worth keeping an eye on possible negative side effects. Does the program create incentives for ever-increasing data collection or other systematized privacy violations that might hurt many other people aside from those helped? Could the compilation of data even about individuals who are helped be stigmatizing, prejudicial, or otherwise harmful to them in other contexts? And of course, as we know only too well, data that is collected for one purpose can easily be turned to other ends.
Next City Daily reports that the data used in this project is all “public”—I don’t know the details of how such information is handled in municipal governments, and it’s not clear to me exactly what that means here. Where a project like this might involve accessing sensitive private data that it would not already have access to in order to help people, that is where the difficult privacy policy questions are to be found.
Accuracy also matters. In this case analytics appear to have been used to focus and guide an already highly discretionary deployment of limited assistance resources; the consequences of an off-base analysis are probably small since even a flawed algorithm is likely to be better than the laborious, much less effective manual process that it replaced. But where inaccurate analytics result in assistance or other benefits being unfairly diverted from people who otherwise would and should have received them, that might be a different story.
Finally, institutional incentives do matter. The contexts where big data is discussed as a potential threat are those where the interests of those doing the data mining are different (sometimes diametrically so) from the interests of the subjects of the data mining. Government security agencies and others using data for “risk assessment” purposes are trying to decide who should be blacklisted, scrutinized, put under privacy-invading investigatory microscopes, or otherwise limited in their freedom and opportunity. Corporate uses of big data can help customers in some ways and hurt them in others, but in the end companies are out to increase their profits and that means squeezing more money out of customers, and that’s usually a zero-sum proposition.
Government social services agencies, on the other hand, have a mission of helping people. One need not be naïve about the shortcomings of government bureaucracies, which as I argued here are best understood as machinery that mindlessly and expansively follow whatever mission they’ve been given. But the nature of that mission is important. While any agency can exhibit the foolishness and irrationalities we associate with bureaucracies, and must be well-managed and subject to checks and balances, it makes a great difference whether bureaucratic machinery has marching orders such as “collect as much information as you can about everyone who could ever possibly be a terrorist,” or “try to minimize our city’s levels of evictions and homelessness.” Some agencies’ missions simply conflict with individual rights and interests much more than others. (Incidentally, this may be the principal argument for a post-Watergate liberal, as opposed to libertarian, attitude toward government.)
Like so many tools, big data analytics can be used for good or for ill. As I have argued, there are very good reasons to think that its consequences may overall be negative. That does not mean it can’t be put to many good uses, however—though even in those cases the devil can lie in the details.